At
the
Everlaw
Summit
in
San
Francisco
last
week,
the
annual
customer
conference
of
the
e-discovery
company
Everlaw,
founder
and
CEO
AJ
Shankar
delivered
a
keynote
address
in
which
he
announced
the
general
availability
of
three
generative
AI
features
the
company
first
introduced
last
year
and
had
been
developing
in
beta
ever
since.
In
the
course
of
delivering
that
address
(see
featured
image
above),
Shankar,
a
computer
scientist
by
training,
detailed
the
core
principles
that
guide
the
company’s
AI
development
–
principles
that
he
said
are
“table
stakes”
to
ensuring
responsible
AI
development
and
the
best
long-term
outcomes
for
customers.
The
three
features
announced,
all
under
the
umbrella
name
Everlaw
AI
Assistant,
are
now
live
on
the
Everlaw
platform,
although
customers
must
purchase
credits
beyond
their
standard
subscriptions
to
use
them.
They
are:
-
Review
Assistant,
for
reviewing,
summarizing
and
prioritizing
documents. -
Coding
Suggestions,
for
coding
and
categorizing
documents
based
on
criteria
provided
by
the
user. -
Writing
Assistant,
for
analyzing
and
brainstorming
against
documents,
evidence
and
depositions.
Three
Core
Principles
At
a
time
when
many
legal
professionals
still
question
the
safety
and
accuracy
of
generative
AI,
it
was
notable
that
Shankar
devoted
a
substantial
portion
of
his
keynote
to
talking
not
about
the
products,
per
se,
but
about
the
three
core
principles
that
guided
their
development
and
Everlaw’s
development
of
other
AI
products
still
to
come.
Those
principles
are:
-
Privacy
and
security. - Control.
- Confidence.
With
regard
to
privacy
and
security,
Shankar
said
that
Everlaw
ensures
that
providers
of
the
large
language
models
it
uses
adhere
to
strict
data
retention
policies.
Everlaw
prevents
LLM
providers
from
storing
any
user
data
beyond
the
immediate
query
and
from
using
that
data
for
model
training.
Keynote
speaker
Shankar
Vedantam,
creator
and
host
of
the
Hidden
Brain
podcast,
is
interviewed
by
journalist
Thuy
Vu.
“We
ensure
that
they
apply
zero
data
retention
to
your
data,
which
means
that
when
you
send
data
to
them,
they’re
not
allowed
to
store
it
for
any
reason
past
when
they’ve
answered
your
query,
as
well
as
no
training,
so
they
can’t
use
the
data
to
train
their
models
in
any
way.”
With
regard
to
control,
Shankar
said
Everlaw
is
committed
to
enabling
users
to
maintain
control
over
their
data
and
tool
usage
through
features
that
allow
them
to
manage
visibility,
access,
and
project-specific
settings.
Everlaw’s
approach
to
transparency
includes
notifying
users
when
they
are
using
AI-powered
features
and
making
it
clear
which
models
are
in
use.
Administrative-level
control
allows
admins
to
control
access
to
AI
features
as
well
as
consumption
of
AI
credits
at
various
organizational
and
project
levels.
“Your
users
should
always
know
when
they’re
using
gen
AI,”
Shankar
said.
“We’ll
tell
you
what
models
we
use.
We
want
you
to
have
that
kind
of
transparency
and
control
in
your
interactions
here,
so
you
can
best
devise
how
to
use
a
tool.”
The
third
principle
–
that
of
enabling
customers
to
have
confidence
in
using
these
tools
–
is
the
hardest,
Shankar
said.
“We
know
gen
AI
can
provide
immense
value,
but
it
can
also
make
mistakes,
right.
We
all
know
about
the
potential
for
so
called
hallucinations.”
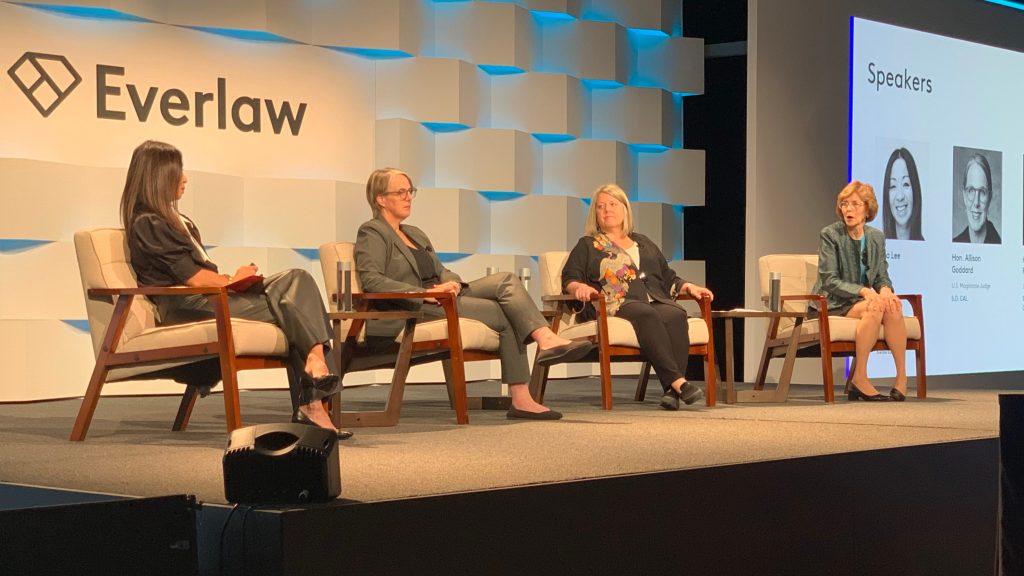
A
panel
of
judges
share
their
perspectives
on
AI,
technology
and
the
law.
From
left:
moderator
Gloria
Lee,
Everlaw’s
chief
legal
officer;
U.S.
Magistrate
Judge
Allison
Goddard
of
the
Southern
District
of
California;
Superior
Court
Judge
Evette
Pennypacker
of
Santa
Clara
County,
Calif.;
and
U.S.
District
Judge
Rebecca
Pallmeyer
of
the
Northern
District
of
Illinois.
Shankar
outlined
two
ways
Everlaw’s
development
of
AI
seeks
to
establish
confidence
in
the
AI’s
results.
-
Play
to
AI’s
strengths.
“The
first
thing
we
do
is
that
we
design
experiences
that
play
to
the
strengths
of
large
language
models
and,
to
the
extent
possible,
avoid
their
weaknesses.”
That
means
focusing
on
use
cases
where
LLMs
have
reliable
innate
capabilities,
such
as
natural
language
fluency,
creativity,
and
even
some
reasoning.
Even
then,
he
said,
“we’re
really
wary.”
For
that
reason,
Everlaw
avoids
uses
that
require
embedded
knowledge
of
the
law
and
instead
delivers
results
that
rely
on
the
four
corners
of
the
document
set
on
which
the
customer
is
working
–
documents
provided
to
the
model
when
it
is
queried,
not
when
it
is
being
trained.
“That
makes
a
far
more
reliable
experience.” -
Embed
into
existing
workflows.
By
embedding
the
AI
into
customers’
existing
workflows,
rather
than
in
a
conversational
chat
interface
that
gives
open-ended
answers,
the
AI
is
able
to
deliver
answers
with
greater
precision.
“We
don’t
want
users
having
to
learn
how
to
prompt
engineer
to
get
what
they
want.
They
basically
will,
in
many
cases,
just
click
a
button
and
we’ve
done
the
work
for
that
precise
use
case
to
ensure
it’s
going
to
be
reliable.”
This
embedding
into
workflows
also
means
that
the
necessary
context
is
provided
to
more
precisely
answer
the
question.
“So,
together,
being
able
to
have
precise
use
cases
and
having
all
the
context
you
need
allows
for
protective
guardrails
and
higher
quality
outputs.”
But
he
said
there
is
a
third
aspect
of
building
confidence
in
the
AI,
and
it
is
something
customers
have
to
do
for
themselves,
which
is
to
change
their
mental
model.
“What
you
basically
have
to
do
is
think
about
using
a
computer
a
little
bit
differently
from
how
we’ve
all
been
trained
to
do
for
many
years.
You
have
to
move
from
an
interaction
model
where
you
have
very
repeatable
interactions
that
are
also
largely
inflexible,
like
a
calculator,
to
a
variable-interactions
model,
where
things
might
be
a
little
different,
but
it’s
highly
flexible.
It’s
much
more
like
a
human.”
‘A
Smart
Intern’
In
fact,
he
urged
the
audience
to
think
of
gen
AI
as
a
“smart
intern”
–
very
capable
and
very
hard
working,
but
still
able
to
make
mistakes.
Over
time,
you
need
to
learn
what
the
intern
is
capable
of
and
determine
your
personal
comfort
level
with
its
capabilities,
but
in
the
meanwhile,
you
need
to
continue
to
check
its
work.
“In
this
new
world,
it’s
neither
good
to
just
blindly
trust
the
output
of
a
gen
AI
tool,
nor
is
it
good
to
just
say,
hey,
one
mistake
and
it’s
out.
It’s
like
a
person,
and
that’s
a
fundamental
shift
in
how
we
want
you
to
think
about
these
tools.”
Just
as
you
would
with
an
intern,
in
order
to
build
confidence
in
the
AI,
you
need
to
check
its
work,
to
learn
what
it
is
good
at
and
what
it
is
not.
For
that
reason,
he
said,
Everlaw
builds
its
AI
products
with
features
that
make
it
easy
for
users
to
check
the
outputs.
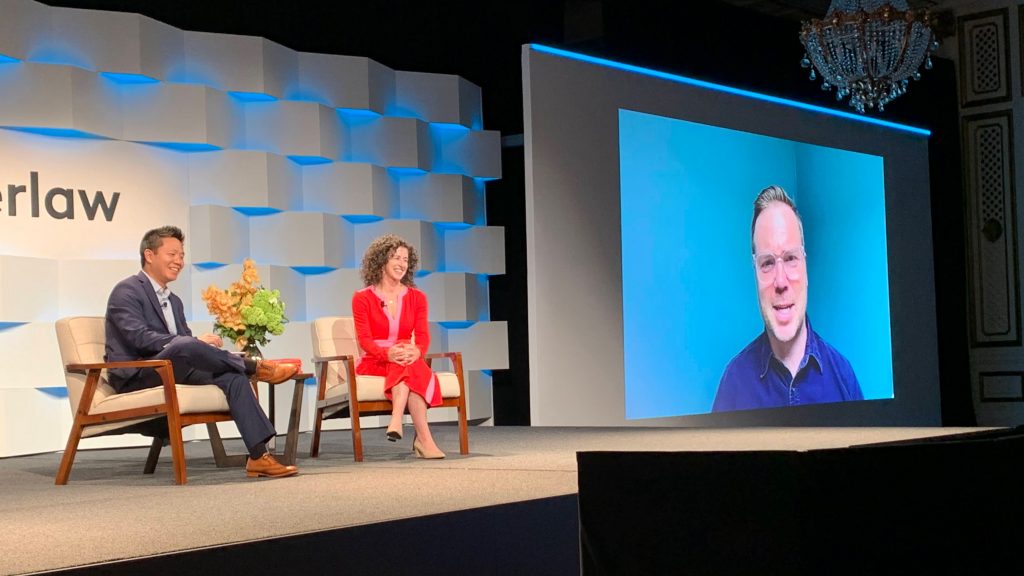
A
virtual
Kevin
Roose,
tech
columnist
for
The
New
York
Times,
is
interviewed
by
Alex
Su,
chief
revenue
officer
at
Latitude,
and
Rachel
Gonzalez,
director
of
customer
marketing
at
Everlaw.
“Our
answers
will
cite
specific
passages
in
a
document
or
specific
documents
when
you’re
looking
at
many
documents
at
once,
and
so
you
can
check
that
work.”
A
specific
example
of
this
ability
to
check
the
AI’s
work
can
be
found
in
the
new
Coding
Suggestions
feature,
which
will
evaluate
and
code
each
document
in
a
set
based
on
instructions
you
provide,
much
like
human
reviewers
would
do.
Unlike
predictive
coding,
it
will
actually
provide
an
explanation
for
why
it
coded
a
document
a
certain
way,
and
cite
back
to
specific
snippets
of
text
within
the
source
document
that
support
its
coding
decisions.
This
allows
the
user
to
quickly
verify
the
results
and
understand
why
the
document
was
coded
as
it
was.
“It
has
a
richer
semantic
understanding
of
the
context
of
each
document,
which
allows
for
a
unique
insight
like
a
human,
potentially
beyond
what
predictive
coding
could
provide
by
itself,”
Shankar
said.
A
Skeptic
Converted
During
his
keynote,
Shankar
invited
onto
the
stage
two
customers
who
had
participated
in
the
beta
testing
of
these
AI
products.
Of
particular
interest
was
customer
Cal
Yeaman,
project
attorney
at
Orrick,
Herrington
&
Sutcliffe,
who
admitted
he
had
been
highly
skeptical
of
using
gen
AI
for
review
before
testing
the
Review
Assistant
and
the
related
Coding
Suggestions
features
for
himself.
In
his
testing,
he
compared
the
results
of
the
gen
AI
review
tool
against
the
results
of
both
human
review
and
predictive
coding
for
finding
responsive
and
privileged
documents.
“I
was
surprised
to
find
that
the
generative
AI
coding
suggestions
were
more
accurate
than
human
review
by
a
statistically
significant
margin,”
he
reported.
He
speculated
that
others
might
get
different
results
when
using
the
gen
AI
review
tool,
depending
on
their
criteria
for
the
case,
the
nature
of
the
case,
and
the
underlying
subject
matter.
“But
the
more
subject
matter
expertise
is
required,
the
more
it’s
going
to
favor
something
like
the
generative
AI
model,”
he
said.
Another
way
in
which
the
gen
AI
review
impressed
him
was
its
consistency
in
coding
documents.
“If
it
was
right,
it
was
consistently
right
the
whole
way
through.
If
it
was
wrong,
it
was
consistently
wrong
the
whole
way
through.”
That
consistency
meant
less
QC
on
the
back
end,
he
said.
He
also
commented
on
the
speed
of
the
gen
AI
tool
compared
to
other
review
options.
In
just
a
few
hours,
he
was
able
to
complete
two
tranches
of
review
of
some
4,000-5,000
documents,
including
privilege
review.
Even
for
someone
who
is
inefficient
in
their
use
of
gen
AI,
the
review
would
have
cost
less
than
half
that
of
a
managed
review,
and
for
someone
who
is
proficient
in
these
tools,
the
cost
would
be
only
5-20%
of
the
cost
of
managed
review.
“So
it
was
a
massive
savings
to
the
client,”
he
said.
Of
course,
cost
doesn’t
matter
if
the
product
can’t
do
the
job,
he
said.
On
this,
he
said,
of
all
the
documents
that
the
model
suggested
were
not
relevant,
the
partner
who
reviewed
the
results
as
the
subject
matter
expert
found
only
one
that
he
considered
was
relevant,
and
that
was
a
lesser-inclusive
email
that
was
already
represented
in
the
production
population.
He
said
it
was
also
highly
impressive
in
its
identification
of
privileged
documents,
catching
several
communications
among
lawyers
who
the
review
team
had
not
been
aware
of
or
who
had
moved
on
to
other
positions.
In
one
instance,
it
flagged
an
email
based
only
on
a
snippet
of
text
that
a
client
had
copied
from
one
email
chain
and
pasted
into
another
email
with
only
the
lawyer’s
first
name
to
identify
him
and
no
reference
to
him
as
an
attorney.
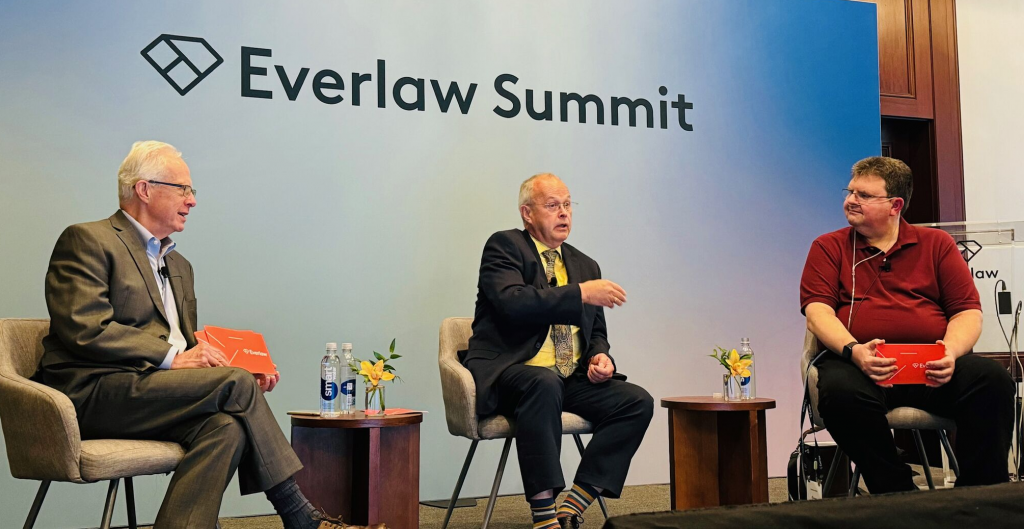
I
moderated
a
panel
on
uncovering
key
evidence
in
high-profile
litigation
with
panelists
Mark
Agombar,
director
of
XBundle
Ltd.,
who
worked
on
the
U.K.’s
Post
Office
Horizon
litigation,
and
Greg
McCullough
of
Fire
Litigation
Consulting,
who
is
currently
working
on
litigation
relating
to
the
Maui
wildfire.
“There’s
no
indication
that
it
was
an
email
to
an
attorney.
There’s
no
indication
that
it’s
necessarily
privileged.
Nothing
in
the
metadata.
No
nothing.”
Overall,
he
said,
there
was
close
alignment
between
the
gen
AI
coding
suggestions
and
the
predictive
coding,
with
their
suggestions
generally
varying
by
no
more
than
5-10%.
However,
in
those
cases
where
there
was
sharp
contrast
between
the
generative
AI
suggestions
and
the
machine
learning
models,
he
said,
then
in
every
instance
the
subject
matter
expert
found
that
the
gen
AI
had
gotten
it
right.
“Those
documents
tended
to
be
something
that
needed
some
sort
of
heuristic
reasoning,
where
you
need
some
sort
of
nuance
to
the
reasoning,”
he
said.
Other
New
Products
For
all
the
focus
on
generative
AI
at
the
Everlaw
Summit,
Shankar
noted
that
only
20%
of
the
company’s
development
budget
is
devoted
to
gen
AI,
with
the
rest
going
to
enhancing
and
developing
other
features
and
products.
In
a
separate
presentation,
two
of
the
company’s
product
leads
gave
an
overview
of
some
of
the
other
top
features
rolled
out
this
year.
They
included:
-
Multi-matter
models
for
predictive
coding.
This
provides
the
ability
to
leverage
predictive
coding
models
created
in
one
matter
to
be
reused
in
subsequent
similar
matters,
making
it
possible
to
generate
prediction
scores
on
new
matters
almost
immediately.
Over
time,
customers
will
be
able
to
create
libraries
of
predictive
coding
models. -
Microsoft
Directory
Integration
for
Legal
holds.
This
feature
allows
users
to
create
dynamic
legal
hold
directories
by
connecting
a
Microsoft
Active
Directory
to
their
legal
holds
on
Everlaw.
That
can
streamline
the
process
of
creating
a
legal
hold
and
keep
custodian
information
in
existing
legal
holds
up
to
date. -
Enhancements
to
Everlaw’s
clustering
and
data
visualization
tools.
A
Note
on
the
Conference
This
was
my
first
time
attending
the
Everlaw
Summit.
As
it
generally
the
case
with
customer
conferences,
there
would
be
little
reason
to
attend
for
those
who
are
not
either
customers
or
considering
becoming
customers.
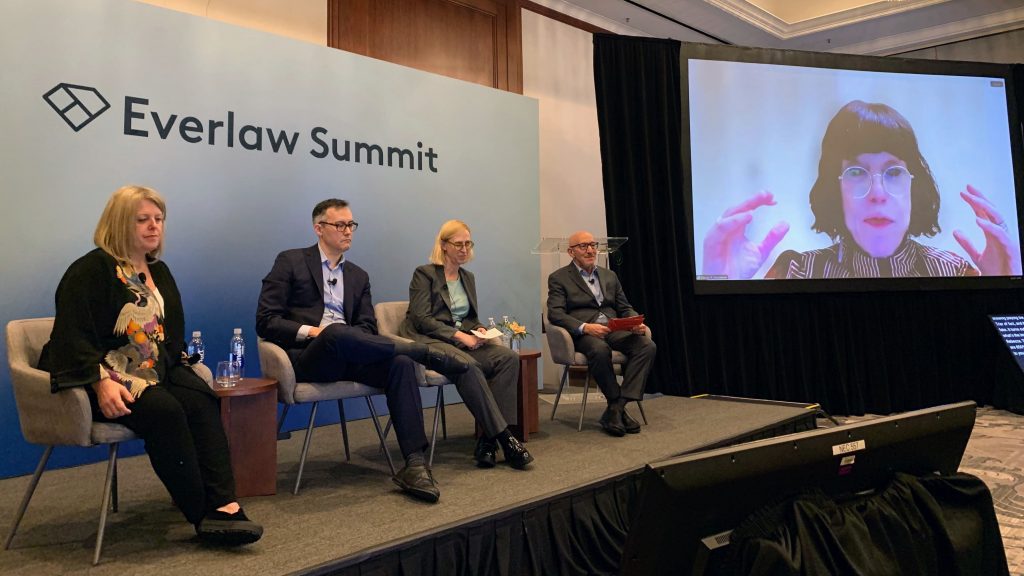
Panelists
who
tackled
the
issue
of
deepfakes
in
the
courtroom
were
Judge
Evette
Pennypacker
from
the
Superior
Court
of
Santa
Clara
County,
Calif.;
Justin
Herring,
partner
at
Mayer
Brown;
Rebecca
Delfino,
associate
dean
at
Loyola
Law
School;
Chuck
Kellner,
strategic
discovery
advisor
at
Everlaw;
and
Maura
Grossman,
research
professor
at
the
University
of
Waterloo.
That
said,
the
more
than
350
attendees
(plus
Everlaw
staff
and
others)
got
their
money’s
worth.
The
programs
that
I
attended
were
substantive
and
interesting,
and
many
covered
issues
that
were
not
product
focused,
but
of
broad
interest
to
legal
professionals.
(I
moderated
one
such
panel,
looking
at
the
discovery
issues
and
strategies
in
two
high-profile
litigations
that
have
been
in
the
news.)
The
conference
also
featured
two
fascinating
“big
name”
speakers
–
Shankar
Vedantam,
creator
and
host
of
the
Hidden
Brain
podcast,
and
Kevin
Roose,
technology
columnist
for
The
New
York
Times.
An
unfortunate
sidebar
to
the
conference
was
the
strike
by
workers
at
The
Palace
Hotel,
the
Marriott-owned
hotel
where
the
conference
was
held.
Just
a
couple
days
before
the
conference
started,
they
started
picketing
outside
the
hotel,
joining
a
strike
and
picket
lines
that
are
ongoing
at
Marriott
hotels
throughout
the
United
States.
Workers
are
seeking
new
collective
bargaining
agreements
providing
higher
wages
and
fair
staffing
levels
and
workloads.
You
can
read
more
about
the
hotel
workers’
campaign
at
UnitedHere!
and
find
hotels
endorsed
by
UniteHere
at
FairHotel.org.